Fair Book Deals
An Introduction to Statistical Learning: with Applications in R (Springer Texts in Statistics) 2nd ed ISBN: 9781071614174
An Introduction to Statistical Learning: with Applications in R (Springer Texts in Statistics) 2nd ed ISBN: 9781071614174
SKU:
Couldn't load pickup availability
- Free & Fast Shipping
- Easy Return
- Secure Payment
Exclusive Offers Just for You!
Save 15% on orders of $399+
Use Code: DEAL15 📋 Copied!
Save 20% on orders of $799+
Use Code: DEAL20 📋 Copied!
✈️ Enjoy Free Shipping on all orders!
Product Details
- Condition: New
- Publisher: Springer
- Language: English
- Paperback: Hardcover
- ISBN: 978-1071614174
- Item Weight: 3.6 pounds
- Dimensions: 7.17 x 1.65 x 10.08 inches
Description
Description
An Introduction to Statistical Learning provides an accessible overview of the field of statistical learning, an essential toolset for making sense of the vast and complex data sets that have emerged in fields ranging from biology to finance to marketing to astrophysics in the past twenty years. This book presents some of the most important modeling and prediction techniques, along with relevant applications. Topics include linear regression, classification, resampling methods, shrinkage approaches, tree-based methods, support vector machines, clustering, deep learning, survival analysis, multiple testing, and more. Color graphics and real-world examples are used to illustrate the methods presented. Since the goal of this textbook is to facilitate the use of these statistical learning techniques by practitioners in science, industry, and other fields, each chapter contains a tutorial on implementing the analyses and methods presented in R, an extremely popular open source statistical software platform.
Two of the authors co-wrote The Elements of Statistical Learning (Hastie, Tibshirani and Friedman, 2nd edition 2009), a popular reference book for statistics and machine learning researchers. An Introduction to Statistical Learning covers many of the same topics, but at a level accessible to a much broader audience. This book is targeted at statisticians and non-statisticians alike who wish to use cutting-edge statistical learning techniques to analyze their data. The text assumes only a previous course in linear regression and no knowledge of matrix algebra.
This Second Edition features new chapters on deep learning, survival analysis, and multiple testing, as well as expanded treatments of naïve Bayes, generalized linear models, Bayesian additive regression trees, and matrix completion. R code has been updated throughout to ensure compatibility.
Four of the authors co-wrote An Introduction to Statistical Learning, With Applications in R(ISLR), which has become a mainstay of undergraduate and graduate classrooms worldwide, as well as an important reference book for data scientists. One of the keys to its success was that each chapter contains a tutorial on implementing the analyses and methods presented in the R scientific computing environment. However, in recent years Python has become a popular language for data science, and there has been increasing demand for a Python-based alternative to ISLR. Hence, this book (ISLP) covers the same materials as ISLR but with labs implemented in Python. These labs will be useful both for Python novices, as well as experienced users.
Shipping, Return & Exchange
Shipping, Return & Exchange
Shipping & Delivery:
- Normal Delivery: Estimated delivery time is 5 to 7 business days from the date of shipment.
- Express Delivery: Estimated delivery time is 3 to 5 business days from the date of shipment.
Returns & Exchange:
- We accept returns within 30 days after delivery. Please read our refer to our Return and Exchange Policy for more detail.
Have a question? Get in touch.
Call or chat with us on whatsapp +1 (302) 329 5375 support@fairbookdeals.comShare
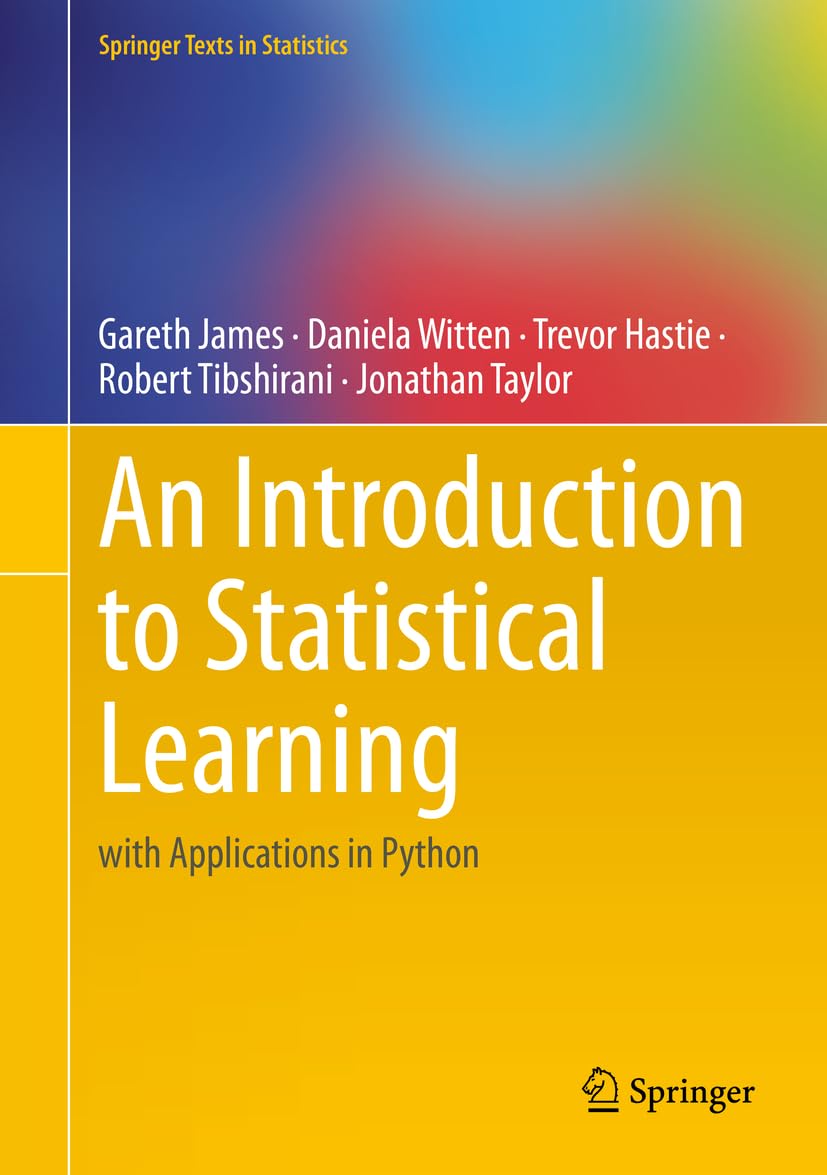
This book makes statistical learning concepts easy to understand and apply. Highly recommended for beginners and intermediate learners.
An excellent introduction to machine learning with clear explanations and practical Python applications.
This book is a valuable reference for both beginners and experienced professionals in statistical learning and data science.
Well-written and highly informative. A great resource for students and professionals interested in statistical learning.
A fantastic guide to statistical learning that covers essential concepts in a simple and engaging way.